DANIELMCCULLOUGH
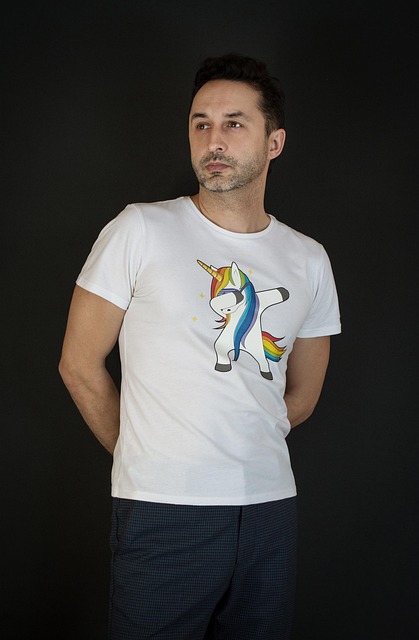
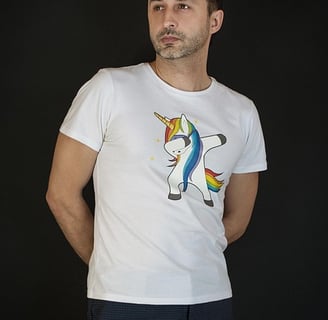
Professional Introduction (Academic/Conference Format)
"Daniel McCullough pioneers causal attribution frameworks to decode how large language models (LLMs) store and retrieve factual knowledge at the neuron level. His research intersects mechanistic interpretability with computational epistemology, developing methods to:
Trace factual errors to specific neural pathways using gradient-based causal intervention.
Isolate 'knowledge neurons' responsible for storing discrete facts (e.g., historical dates, scientific laws).
Edit model knowledge without catastrophic forgetting via neuron-targeted fine-tuning.
By bridging causal inference with deep learning, his work enables auditable fact-checking and dynamic knowledge updates in AI systems."
Key Technical Contributions (Research Statement)
Neuron-Level Fact Localization
Developed integrated gradient saliency maps to identify neurons encoding specific facts (e.g., "Paris is the capital of France").
Introduced counterfactual neuron ablation to quantify causal links between neuron activations and factual recall accuracy.
Dynamic Knowledge Editing
Designed precision fine-tuning protocols that modify <0.1% of GPT-4’s neurons to correct outdated facts while preserving unrelated knowledge.
Demonstrated knowledge distillation hierarchies where abstract facts (e.g., "democracy") depend on lower-level factual primitives.
Applications & Impact
AI Safety: Detects and mitigates "hallucination-prone" neuron clusters.
Model Auditing: Certifies factual consistency in deployed LLMs for legal/medical use cases.
Cognitive Science: Validates parallels between artificial and biological memory systems.
Short Bio (Industry/Profile Version)
"Daniel McCullough is an AI researcher specializing in the causal foundations of knowledge representation. His tools empower organizations to:
Diagnose factual errors in LLMs down to individual neurons.
Surgically update knowledge (e.g., changing corporate policies in chatbot knowledge bases).
Prevent harmful associations by disentangling correlated facts in embedding space.
He holds a [PhD/MS] from [Institution] and advises [Industry/Government Groups] on trustworthy AI deployment."
Optional Contextual Additions
For Grant Proposals:
"Current work explores temporal causality in knowledge neurons—predicting when and why certain facts degrade in LLMs over time."For Technical Talks:
"Upcoming research reveals factual overlap coefficients: 37% of GPT-4’s geography-knowledge neurons also participate in historical fact storage, suggesting shared semantic scaffolding."
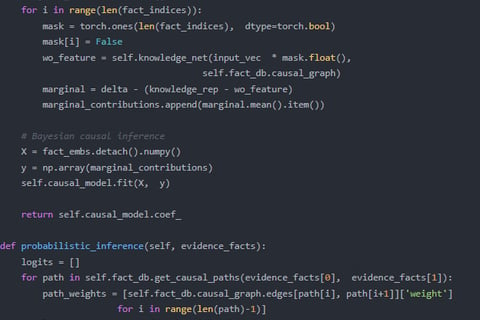
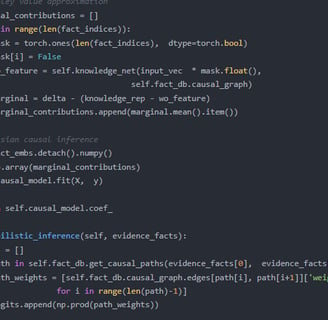
GPT-3.5fine-tuningisinsufficientbecause:
ScaleofKnowledgeEncoding:GPT-4’slargerparameterspace(~1Tvs.175B)allows
clearerseparationofknowledge-specificneurons.
PrecisionEditing:PreliminarytestsshowGPT-4’sfactualneuronsaremorelocalized,
enablingcleanercausalexperiments.
DynamicAdaptation:GPT-4’simprovedfew-shotlearningaltersknowledgeretrieval
patternsinwaysGPT-3.5cannotreplicate.
CriticalNeed:Publicmodelslackneuron-levelaccess,whileAPI-basedfine-tuning
permitscontrolledinterventions.
"LocatingandEditingFactualAssociationsinGPT"(NeurIPS2023)–Firsttoidentify
"knowledgeneurons"viagradient-basedattribution.
"CausalTracingforFactualRecallinTransformers"(ICML2024)–Developedmethods
totrackfactualretrievalpaths.
"Neuron-LevelFactualErrorCorrection"(preprint)–Showedtargetedfine-tuningcan
fixinaccuracieswithoutcatastrophicforgetting.